The promise of artificial intelligence is everywhere today. From self-driving cars to robotic companions for the elderly, technology’s ability to mimic human intelligence is creating targeted algorithms that solve puzzles, recognize faces, respond to verbal commands and perform far more complex tasks. One example: algorithms that can accurately and predictably diagnose disease, in some cases far better than doctors can.
But artificial intelligence isn’t infallible. And it carries with it a veritable Pandora’s box, the contents of which we have yet to discern. In fact, at a Conference on Precision Medicine in Boston this week, Harvard Law School professor Jonathan Zittrain likened AI in healthcare to asbestos:
“It turns out that it’s all over the place, even though at no point did you explicitly install it, and it has possibly some latent bad effects that you might regret later after it’s already too hard to get it all out,” he said.
One major issue with AI is its fallibility, Zittrain claims. One example: In 2017, a team at MIT tricked Google’s image recognition software into misidentifying dozens of images using a technique called “adversarial examples.” Simply by tweaking a couple of pixels in an image, which remained unchanged to the human eye, they convinced the software that a turtle was a firearm, a baseball was a cup of espresso, and a cat was guacamole — all with a stated probability of 99.9 percent.
Just imagine the consequences if such mistakes occurred in an algorithm meant to identify disease. As Zittrain remarked, “How do you feel when the [algorithm] spits out with 100 percent confidence that guacamole is what you need to cure what ails you?”
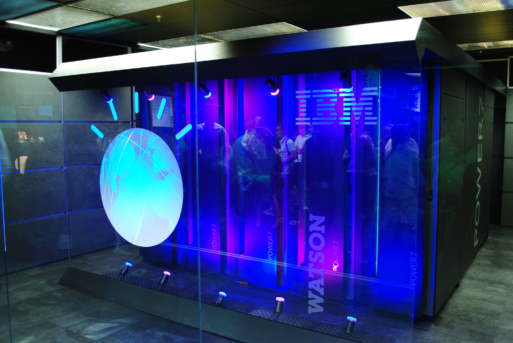
IBM’s Watson was one of the earliest supercomputers capable of machine learning and artificial intelligence
Artificial intelligence also has a tendency to confuse correlation with causation, Zittrain said. AI’s ability to sort through billions of pieces of data allows it to make correlations that the human brain would miss. But many of these correlations are just that — meaningless associations that have no bearing on the disease at hand. (Zittrain points to one such correlation between suicide by hanging in North Carolina and the number of lawyers in the state.)
The risk here, he says, is that doctors who falsely assume these correlations point to causality could make recommendations for care that won’t help the patient and actually may cause harm.
Privacy Concerns
Another major issue with AI is that much of the data collected to create digital therapeutics cannot be anonymized. Unlike medical records, which can be scrubbed clean of identifying information, data from wearables and other nascent technology is unique to the person wearing it. According to Andy Caravos, CEO of Elektra labs, a company that collects data to improve clinical trials, the data coming from wearables is like a digital fingerprint. “I am uniquely identifiable with 30 seconds of walk data,” she said.
Anonymizing a person’s genome is equally problematic, if not downright impossible. Yet researchers are now using AI to create “hyper-individualized therapy” using a person’s genome to determine what treatment is the best fit. This data is collected and analyzed by health tech companies operating virtually free of regulatory oversight, Caravos said. She then floated the idea of treating algorithms and the data used to create them just like drugs. “If you think about digital therapeutics, they all have a certain mechanism of action,” she said. “Is there an argument, with what we’ve learned in health care, to look at [digital treatments] in the same way we look at drugs?”
Inherent Bias
An argument often used to support the use of artificial intelligence in the healthcare setting is that it can eliminate unconscious bias and lead to better quality, standardized care. Because artificial intelligence is generated by a machine that makes decisions based solely on data, it has no unconscious stereotypes or preconceptions that can influence results.
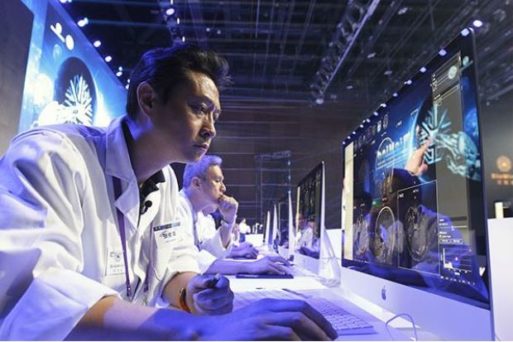
Elite doctors in Beijing pitted their diagnostic skills against artificial intelligence and lost
Credit: China Times
But in the real world, that’s not quite true because data itself is only as reliable as its source. In dermatology, for example, AI is exceptionally skilled at identifying skin cancers — performing better in one study than 58 dermatologists who were part of the study group. But, like human doctors, AI is far less able to identify skin cancers in people who are not white. That’s because most of the data used to train the machines were gathered from patients in the United States, Europe and Australia who had fair skin.
“If you don’t teach the algorithm with a diverse set of images, then that algorithm won’t work out in the public that is diverse,” said Adewole Adamson of the University of Texas at Austin. “So there’s risk, then, for people with skin of color to fall through the cracks,” he added.
Notably, people of color have a lower incidence of skin cancer than fair skinned people. But they have a higher risk of dying from the disease. According to the American Academy of Dermatology, the five-year survival rates for skin cancer in African Americans is 73 percent, versus 90 percent in whites.
Collaboration Is Key
Obviously, artificial intelligence is the wave of the future. We can’t put the genie back in the bottle, no matter how hard we try. But understanding the pitfalls inherent in machine learning and working to correct them is critical to ensuring that AI is used to benefit all humankind. Putting too much faith in technology too soon could be a very dangerous thing.